Dimension Reduction and Graphical Models using Optimal Transport
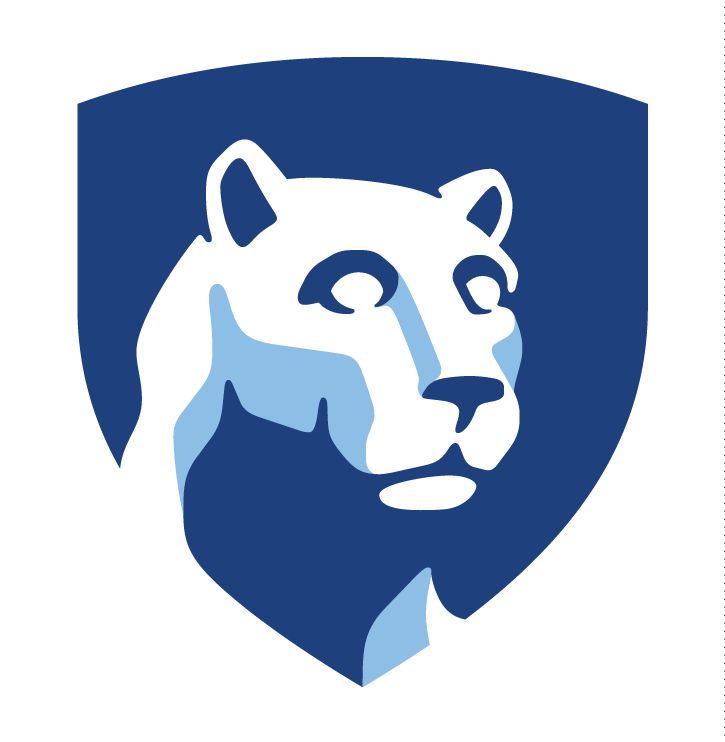
Restricted (Penn State Only)
- Author:
- Zhang, Qi
- Graduate Program:
- Statistics (PHD)
- Degree:
- Doctor of Philosophy
- Document Type:
- Dissertation
- Date of Defense:
- May 15, 2023
- Committee Members:
- Bing Li, Professor in Charge/Director of Graduate Studies
Lingzhou Xue, Co-Chair & Dissertation Advisor
Ting He, Outside Unit & Field Member
Bing Li, Co-Chair & Dissertation Advisor
Runze Li, Major Field Member - Keywords:
- Sufficient dimension reduction;
Metric space-valued data;
Graphical model;
Optimal transport; - Abstract:
- In this dissertation, we explore three topics at the intersection of optimal transport and statistical models, with a focus on dimension reduction and graphical models. In Chapters 3 and 4, we propose novel linear and nonlinear sufficient dimension reduction methods to incorporate distributional objects by analyzing them in metric spaces induced by optimal transport. In Chapter 5, we develop new copula graphical models for multi-attribute data by leveraging the geometry features of the optimal transport map. Below are summaries for each chapter. In Chapter 3, we introduce a flexible linear sufficient dimension reduction (SDR) method for Fréchet regression, where the predictor is modeled in a Euclidean space, and the response object is modeled in a metric space. The framework works for an important case where distributional objects endowed with the Wasserstein metric are treated as the response. The motivation to consider dimension reduction under this setting includes: mitigating the curse of dimensionality caused by high-dimensional predictors and providing a visual inspection tool for regression diagnostics. The basic idea is to first map the metric-space-valued response to a real-valued random variable using a class of functions and then perform classical SDR to the transformed data. Therefore, our approach can turn any existing SDR method for Euclidean data into one for Fréchet regression. The finite-sample performance of the proposed methods is illustrated through simulation studies, and the data visualization aspect is illustrated using the human mortality distribution data. In Chapter 4, we propose a new framework of nonlinear sufficient dimension reduction for cases where both the predictor and the response are distributional data. Our key step is also to build universal kernels on the space of measures, which results in reproducing kernel Hilbert spaces (RKHS) for the predictor and response that are rich enough to characterize conditional independence. We use the Wasserstein distance for univariate distributions, while for multivariate distributions, we resort to the sliced Wasserstein distance. This choice ensures that the metric space possesses similar topological properties to the Wasserstein space while also keeping the negative type of the metric and offering significant computation benefits. Numerical results based on synthetic data show that our method outperforms possible competing methods. The method is applied to several data sets, including fertility and mortality data and Calgary temperature data. In Chapter 5, we propose a novel copula model, called cyclically monotone copula, to relax the Gaussian assumption in the multi-attribute graphical model, which estimates the graph with an edge set that encodes the conditional dependence between vectors. The new copula can efficiently link vector marginals based on the optimal transport theory. The model is more flexible than the classical Gaussian copula model that performs coordinatewise Gaussianization. We establish the concentration inequalities of the estimated covariance matrices and provide conditions for selection consistency using the group graphical lasso estimator. For the setting with high-dimensional attributes, a projected cyclically monotone copula model is proposed to address the curse of dimensionality issues that arise from solving high-dimensional optimal transport problems. We show numerical results based on synthetic data and provide illustrative applications on gene and protein regulatory networks and color texture image data.